Predicting the relationship between a molecule’s structure and its odor remains a difficult. This problem, termed quantitative structure-odor relationship (QSOR) modeling, is an important challenge in chemistry, with a strong potential impact on the manufacture of synthetic fragrance, among others.
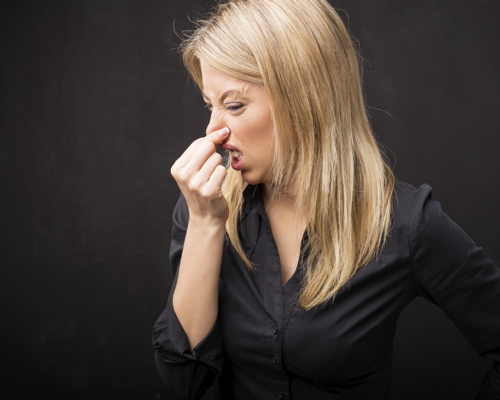
A team of Google researchers recently published a paper on how they trained a neural network to connect a molecule’s structure with its odor. (Photo: © grinvalds/Istock.com)
A paper published on October 23, 2019, by a team of Google researchers tackles how machine learning can be used to find the relationship - which scientists have been trying to quantify for over 70 years - between a molecule’s odor and its structure.
While molecular structures can give scientists insight as to what something looks like or what it sounds like, "predicting the relationship between a molecule’s structure and its odor remains a difficult, decades-old task."
These scientists used machine learning to find this underlying quantitative relationship in a way reminiscent of how deep learning is used to predict visual and auditory characteristics.
Using a dataset of 5030 molecules labeled with odor descriptors (like fruity, bready, nutty and cheese) by olfactory experts (like professional perfumers), the team trained a Graph Neural Network to predict these labels based on the molecule’s shape. The perceptual and structural similarities of both local and global components of a molecule aided this neural network in recognizing which parts are responsible for which fragrance nodes.
According to the paper, being able to use machine learning to predict odors based on their molecular structures would "aid in the discovery of new synthetic odorants" so that natural products don’t need to be harvested in such high numbers.
The latest trends and innovations in fragrance will be presented on November 7, 2019 at the Fragrance Innovation Summit in Paris. Program and registration: www.fragranceinnovation.com |